Kalinin Collaboration Moves Microscopy into the Automated Fast Lane
Vol researchers recently developed a better way to take a closer look, and earned a 2023 R&D100 Award for their success.
Sergei Kalinin, UT’s Weston Fulton Professor in materials science and engineering, and a team of UT and Oak Ridge National Laboratory (ORNL) colleagues earned the award with an innovative project that demonstrated a physics-informed, active learning (AL) driven autonomous microscopy.
Their technique enables active, autonomous discovery of physics during real-time experiments. It can be applied to any microscopic technique and can be adapted for application in other experiments, such as chemical syntheses and battery lifetime testing.
“This involved over three years of teamwork to make it happen,” said Kalinin, co-lead along with ORNL’s Yongtao Liu, Kevin Roccapriore, and Maxim Ziatdinov. “It included code-based development by Ziatdinov, deployment on scanning probe microscopes by Liu and Rama Vasudevan, deployment on scanning transmission electron microscopes by Roccapriore, and SPM integration efforts by Kyle Kelley and Stephen Jesse.”
This marks Kalinin’s fifth R&D100 award. Other milestones within the research for “Physics-Informed, Active Learning–Driven Autonomous Microscopy for Science Discovery” involved two filed patents, more than 15 publications and preprints, and thousands of hours of coding, workflow design, testing, deployment, data analysis, and brainstorming.
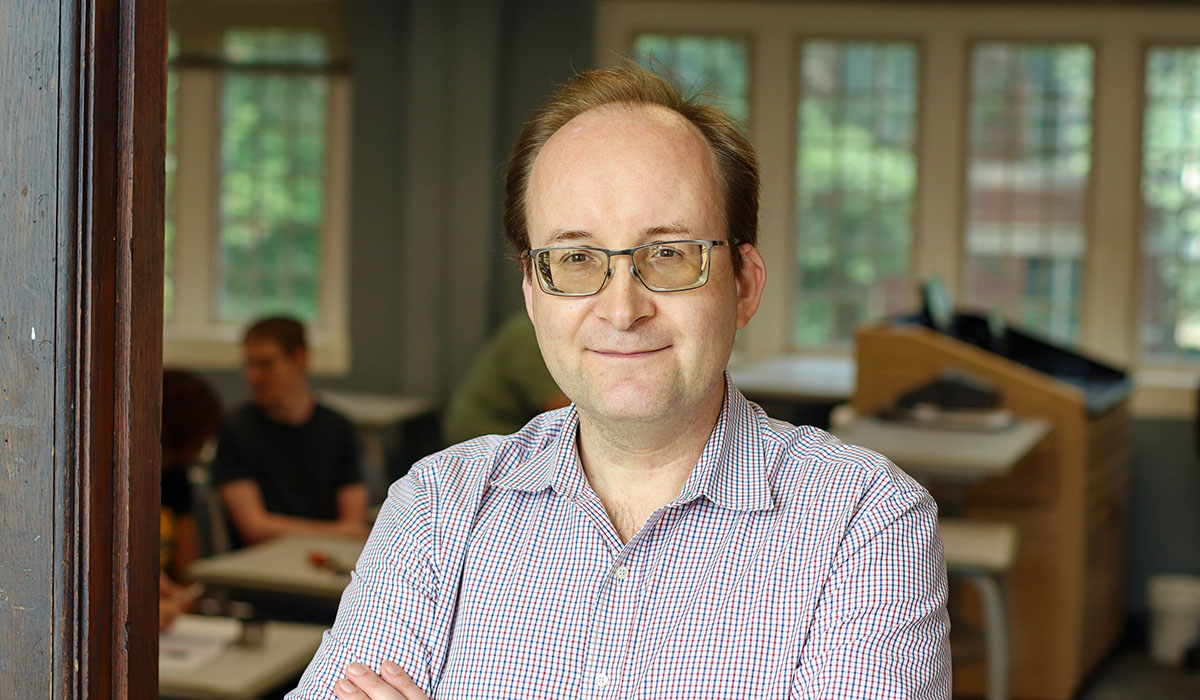
“I’m proud to be a part of the team,” said Kalinin. “Also, we give special thanks to US Department of Energy (DOE) BES for the support of this work via the 3DFEM EFRC center led by Susan Trolier-McKinstry of Penn State.”
In their abstract for the project, the team points out that making microscopes automated and autonomous is a “North Star goal” for disciplines ranging from physics and chemistry to biology and materials science. The aspiration is that applications could discover structure-property relationships, explore physics of nanoscale systems, and build matter on nanometer and atomic scales. This required developing task-specific machine learning (ML) methods, understanding the relationship between physics discovery and ML, and establishing well-defined workflows.
“We can fundamentally change the way microscopy works,” said Kalinin. “Currently, microscopes use rectangular scanning as a result of double technological debt. It is easy to implement and convenient for humans to perceive. However, the objects of interest are not uniform in space—they are usually manifest in a small number of locations. These locations are different depending on our goal. If we want to understand mechanical properties, we look at dislocations, and if we want to understand corrosion at grain boundaries. Currently, we explore everywhere. AI-driven microscopes will discover based on our experimental objectives.”
The group began working on autonomous experimentation in 2019 using the concept of Gaussian processes.
“It was a lot of effort to develop it—at that time Maxim had to write his own implementation—and with codes Rama started to work on control interfaces to deploy it on operational microscope,” said Kalinin. “Around the time when deployment became possible, we started to realize that this algorithm is absolutely insufficient for the goal.”
They turned to a more complex algorithm, deep-kernel learning (DKL), to solve the issue. The DKL code development was led by Ziatdinov and allowed them to overcome the limitations of the simple Gaussian processes. DKL not only worked but is a first example of a beyond-human workflow for microscopy—it can perform tasks that a human running the microscope cannot.
“That was very interesting feeling,” said Kalinin. “Usually, we think about ML as doing same tasks as human, but in automated way. This was a new task.”
The team also came to realize that while fully automated microscopy is unlikely to happen, with a realistic path forward being a human-in-the-loop approach.
“The transition from the human-driven to human-in-the loop, ML-driven microscopy will be not a transition from riding a Toyota to driving a Lamborghini,” said Kalinin. “It will be more like a transition from riding a horse to driving a car—a totally different way to interact with the instrument and do research.”
Contact
Randall Brown (865-974-0533, rbrown73@utk.edu)